Feature Store
Centralize the entire feature lifecycle and focus on building and delivering features in production
Get Started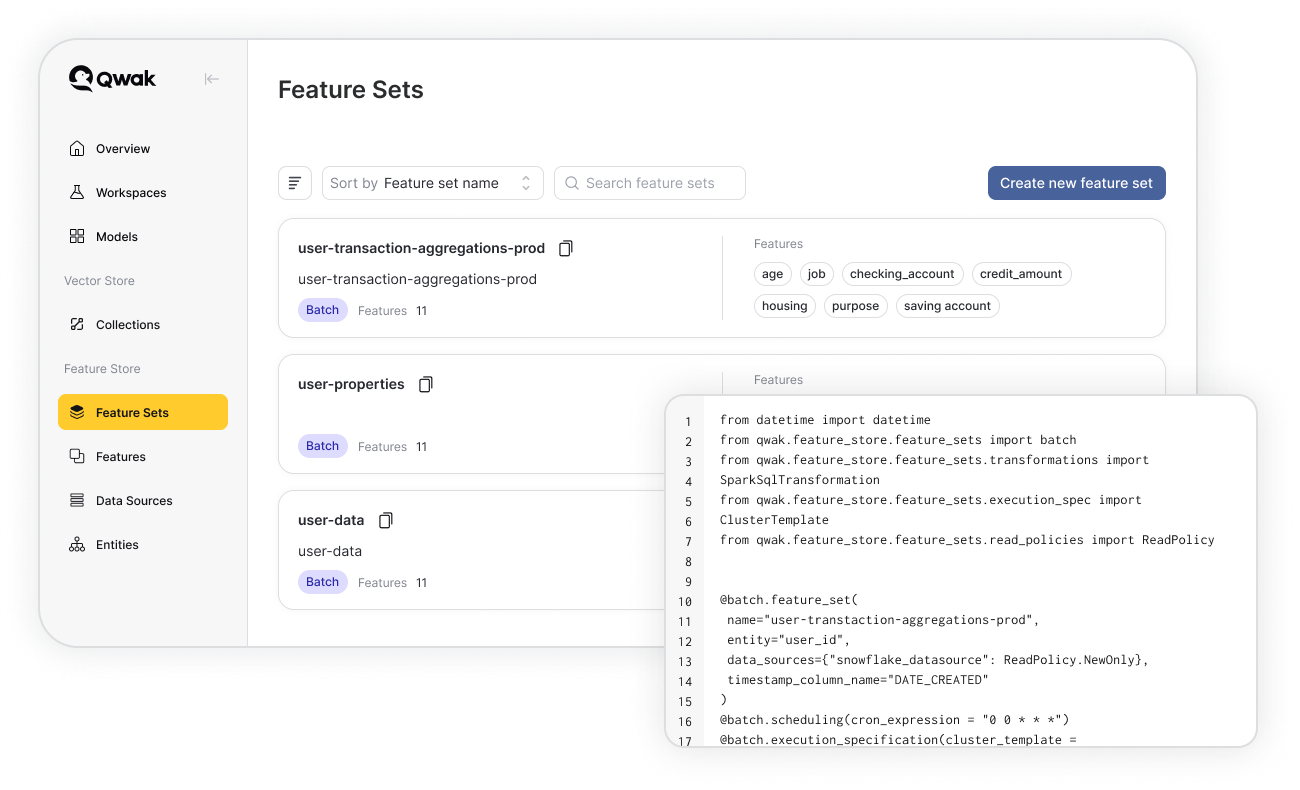
Enable data scientists and ML engineers to easily collaborate and share features across projects.
Ensures the consistency of online and offline generated features.
Serves as a single, discoverable source of truth for features used by production models.
Use Cases
Transform Data
Streamline the creation of features including orchestration and processing of data pipelines. Use a unified system to define transformations across various data sources while.
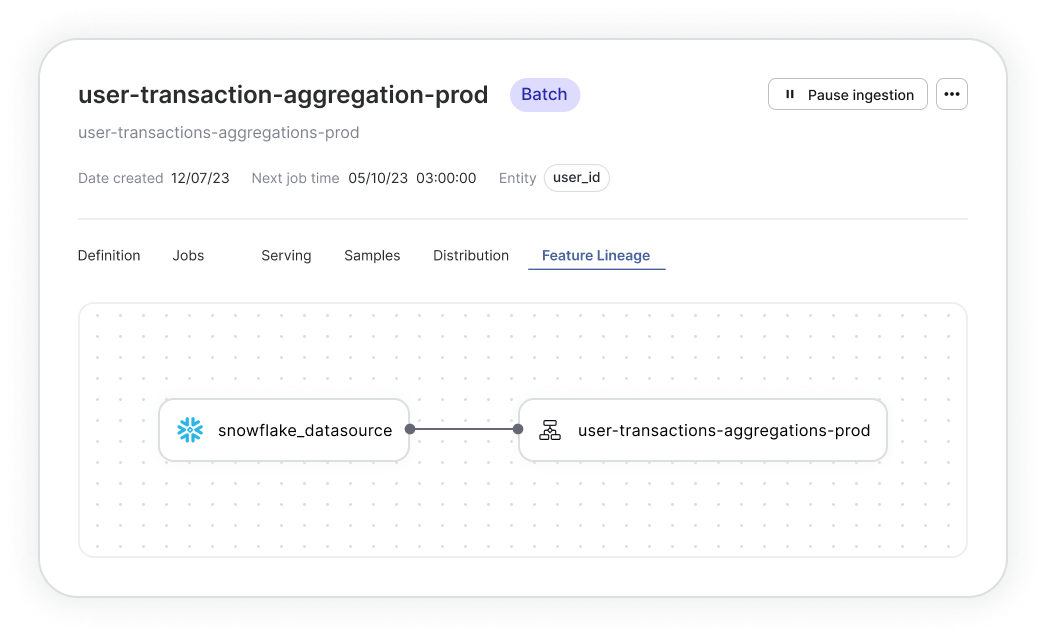
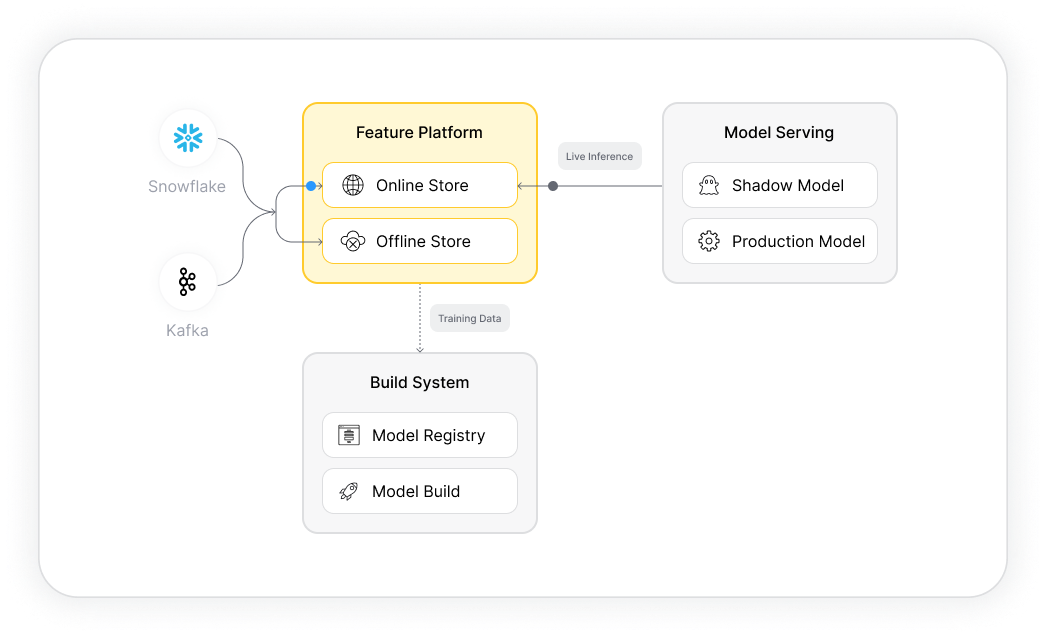
Store Features
Large-scale and cost-effective offline store for training data and an lightning-fast, low-latency online store for inference data access during online serving.
Serve Features
Fast, simple and reliable feature serving for training and inference. Generate high-quality training data and automatically fill in missing features in your training sets.


Data ingestion
1. The feature store ingests data, primarily stored in a data warehouse, and processes it to extract and transform relevant features, and stores these resulting features in a feature store database.
2. Ingest and manage features from multiple data sources, such as structured databases, unstructured logs, text files, and real-time data streams.
3. Continuously collect processing data as it is generated directly from the stream to compute aggregative value (i.e. sum, average, count). Use this process to perform real-time analytics on streaming data or update running aggregate values as new data comes in.
Feature extraction
1. Training API: Store and manage historical versions of features that have been computed and stored in this part of the feature store. Use this API to retrieve historical data for training and testing purposes.
2. Serving API: Real-time serving in a feature store enables the use of the most up-to-date versions of pre-computed features in real-time as they are being generated or updated. This is primarily designed for production use, and can support large scale, high throughput, and low latency. Ideal for applications such as real-time personalization, fraud detection, anomaly detection and more.
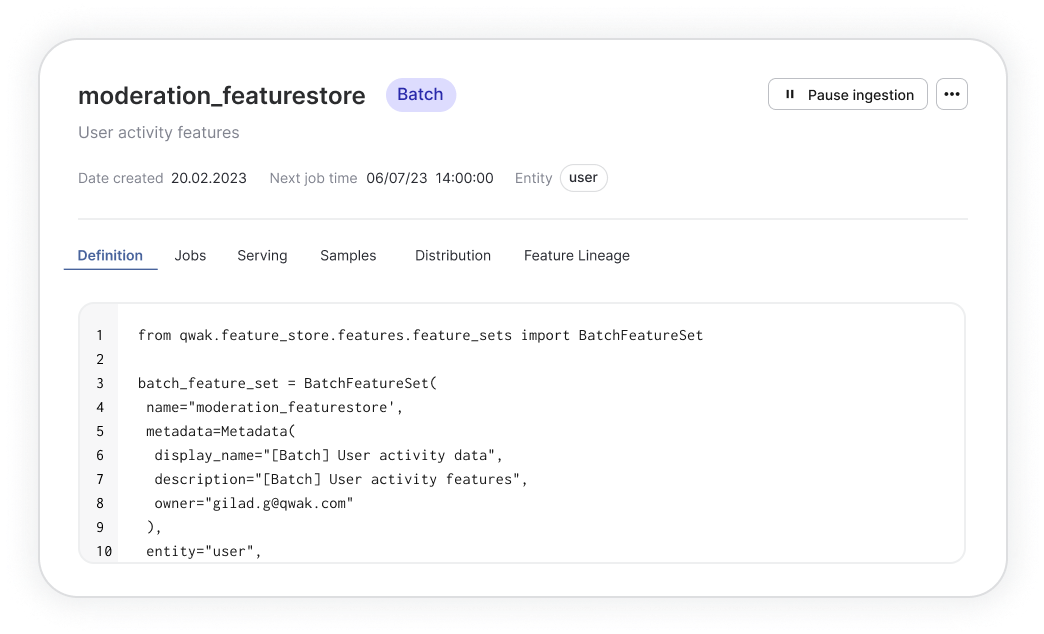
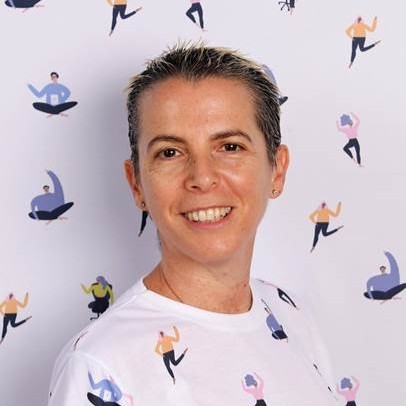
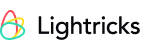
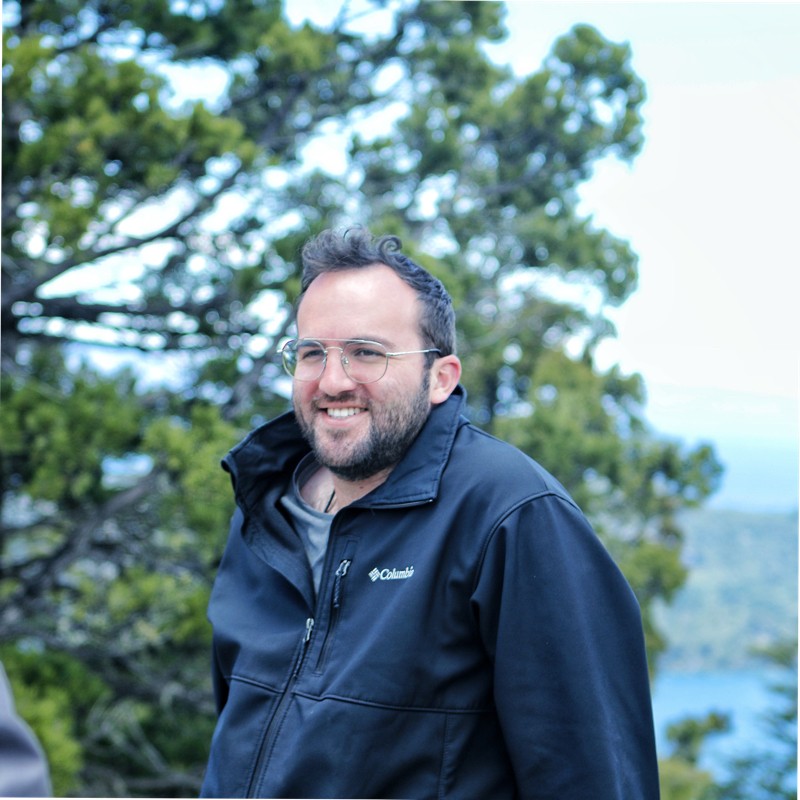

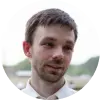
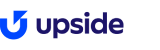