Guesty: Property management platform delivers a game changing RAG-based chatbot
Guesty is an end-to-end platform for property managers and management companies, offering cloud-based tools to simplify operational tasks such as tracking guest check-ins and property revenue, addressing the complex needs of short-term and vacation rentals.
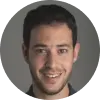
Challenges
The journey of integrating a RAG-based chatbot using LLMs presented Guesty with a set of unique challenges:
- Updating Model Tech Stack: The team got the challenge of deploying a RAG model for the first time, navigating the complexities associated with leveraging LLMs in different areas of their business.
- Lack of a Vector Database: The absence of an existing vector database meant that Guesty needed a reliable solution that could handle the complexities of vector storage and retrieval with low latency requirements.
- Scalability of the Solution: A notable challenge was ensuring the scalability of the chatbot solution. Guesty required a system that could easily transition from a 'low scale' proof of concept to a 'high scale' deployment, catering to an increasing number of customers.Â
To address these challenges, Guesty collaborated with the Qwak team, to enable the vector database technology which offers the required infrastructure and expertise. Qwak's solutions were specifically designed to be user-friendly, allowing Guesty's data science team to lead the implementation with minimal assistance from engineering.
Implementation
The Implementation Journey - Technical Deep Dive
In order to implement the new model, the team created the following process:
.jpeg)
- Resource Data Synthesis: The chatbot sources information from previous guest conversations, property characteristics, and user-saved replies to construct a robust knowledge base saved in Google BigQuery.
- Data Pre-Processing: Prior to vector embedding, resource data is pre-processed for optimal compatibility with the AI model.
- Embedding Generation via OpenAI: OpenAI's algorithms are employed to transform pre-processed data into dense vector embeddings, capturing the nuanced semantics of the text.
- Vector Database: Qwak's Vector DB is leveraged to store and manage these embeddings, optimizing for rapid similarity searches and retrieval.
- Continual Data Enrichment: The database undergoes daily updates of 50,000 rows to incorporate new interactions, constantly evolving the chatbot's response accuracy.
User Query Resolution Framework
- Vectorized Query Processing: User queries are vectorized following the same protocol as the resource data, ensuring consistency in response quality.
- Retrieval Mechanism: The Vector DB conducts a similarity search to retrieve the most relevant vectors corresponding to the user query.
- AI-Powered Response Formulation: Utilizing ChatGPT 3.5's contextual prowess, the chatbot proposes suggested answers to the hosts.
- Tailored Response Generation: A specialized prompt function is employed to craft the final guest response, drawing on the suggested answers and ensuring relevance and personalization.
Solutions
The strategic alliance between Guesty and Qwak delivered significant results in a short time-frame:
- Quick Rollout: The chatbot was up and running in just three weeks, a testament to Qwak's straightforward tools and the know-how of the data science team.
- Data Science-Led Implementation: The deployment was predominantly executed by data scientists, with minimal need for engineering support enhancing the user-friendliness and autonomy enabled by Qwak's solutions.
- Enhanced Operational Efficiency: Qwak's managed vector database and serving capabilities facilitated immediate improvements in response time, directly impacting operational efficiency.
- Cost Savings and Guest Satisfaction: The automated system led to cost reductions and elevated guest service quality, as evidenced by the fast and accurate responses.
- SLA Performance: Hosts experienced a notable uptick in their ability to fulfill SLA commitments, thanks to the chatbot's efficiency.
- Scalability: the transition from POC to Live production was achieved with just one click, showcasing the flexibility and scalability of the Qwak platform. This feature was crucial, as it meant that the solution could be quickly adapted to meet growing demand without the need for extensive reconfiguration or downtime.
Based on this architecture, Guesty plans to ship 2 more models to help improve customer support and internal engineering efficiency.
The chatbot deployment has led to an increase in user engagement, with usage rates rising from 5.46% to 15.78%. This growth indicates a positive reception and increased utilization of the chatbot among users. Alongside this, there's been an improvement in user satisfaction. Users have generally reported satisfaction with the chatbot's responses, finding them accurate and useful for their interactions. This feedback suggests that the chatbot is successfully meeting the needs of its users, aiding in more effective guest communication.