MLOps 2.0: The Next Generation of Machine Learning Operations
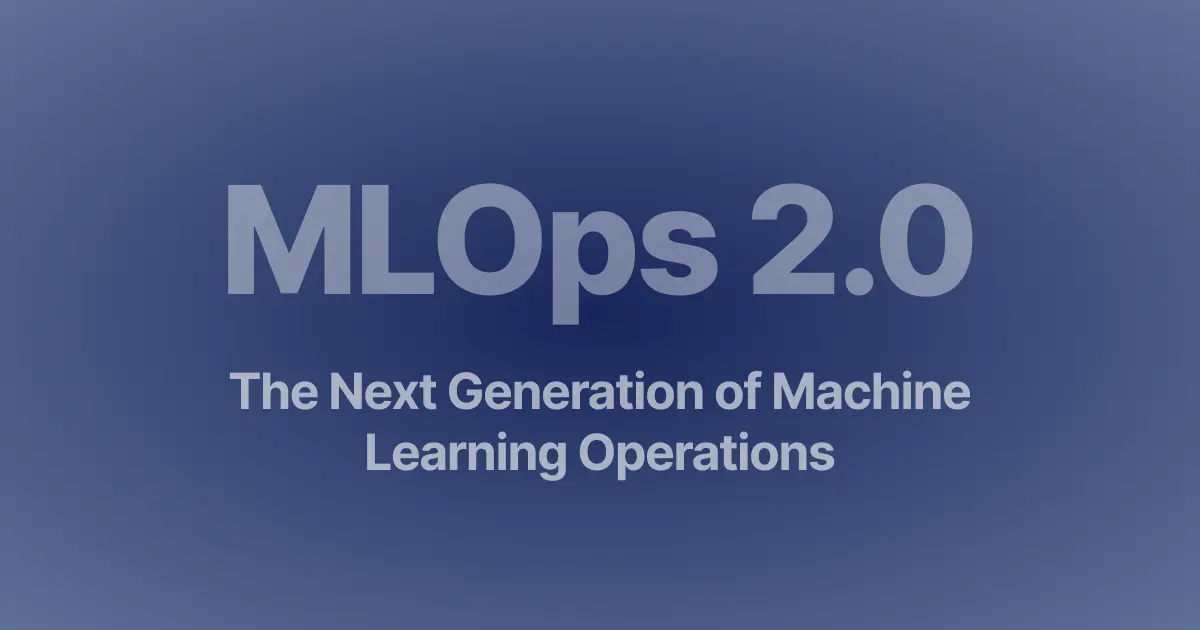
Machine learning (ML) has been transforming industries for years. One of its biggest challenges has always been deploying machine learning models into production environments.Ā
MLOps (Machine Learning Operations) emerged as a solution to these challenges.Ā
The first āgenerationā of MLOps focused on creating experiments and building experimental environments for data scientists. Most models still do not make it into production and get stuck at the experimental stage.
Gartner reports that only half of machine learning models ever make it into production.
MLOps 2.0 shifts the focus and helps organizations adopt a production mindset.
MLOps 2.0, is focused on creating an infrastructure that can support the deployment of machine learning models at scale. This infrastructure should include a feature store, model repository, model serving, model monitoring, and an overall orchestrator to manage everything centrally.
The feature store should handle feature transformation for not only training but simultaneously it should also support production. It enables data scientists to easily define and manage features for their models, and makes it simple to share these features across teams. With a feature store, data scientists can focus on developing models, while the feature store handles feature engineering.
The model repository in MLOps 2.0 should ensure that models are always ready to deploy and reproducible. This allows teams to quickly deploy models and make changes as necessary. The model repository is also versioned, making it easy to track changes over time.
Model serving is a critical component of MLOps 2.0. It allows for high scale while allowing deployment strategies such as shadow deployment, A/B testing, multi-arm, and more. With model serving, teams can easily deploy and monitor models in production environments.
Model monitoring is another important feature of MLOps 2.0. With live model data, teams can monitor and optimize their models in real-time. This allows teams to quickly identify and address issues with their models, improving overall performance and accuracy.
Finally, an orchestrator in MLOps 2.0 allows teams to build retraining mechanisms as part of the process to ensure that models are always up-to-date and can quickly adapt to changing conditions.
With a focus on scalability, reproducibility, and real-time monitoring, MLOps 2.0 enables teams to deploy and manage machine learning models in production environments with ease. As machine learning continues to transform industries, MLOps 2.0 will play an important role in ensuring that these models are deployed and managed effectively.