Building vs buying your machine learning platform

As organizations embrace the potential of data science and machine learning (ML), they face the huge challenge of operationalizing these processes in their technical environments and picking the right investments at the right times according to their strategic objectives.
This raises a big question for decision-makers: Is it better to build a data science platform in-house or to purchase one from a third-party vendor?
Build vs Buy
There’s no doubt that third-party tools and platforms have become a crucial part of how businesses accomplish data management and model development. But the question that often arises it should the platforms be built from the ground up internally or bought from third-party service providers.Â
Although it’s true that many organizations today have highly skilled professionals who have the capability of building solutions from scratch, there are pros and cons to both approaches. The dilemma often boils down to deciding between paying licensing fees for pre-built software platforms or investing in an internal team that will create custom software to solve specific organizational issues.Â
There are lots of factors that come into play when making this decision, including:
- Cost — This is naturally one of the most important determining factors. It’s often the case that building your own tooling from scratch is more expensive than simply buying or licensing one.
- Time — Developing, designing, and deploying a custom platform can take a huge amount of time, and this might cause a loss of opportunities in conducting other important tasks. In contrast, deploying a ready-made solution is often easy and hassle-free.
- Adding features — Data science and ML platform providers are constantly updating their products to add new features and functionality. This is an obvious benefit over building your own platform, where bringing iterations at a faster pace can be quite challenging.
- Flexibility — When you use a third-party tool or platform, there’s a chance that it might not integrate with the other tools used by your team. In contrast, a platform that you’ve built yourself can be completely aligned with what your organization needs.Â
Building vs buying a machine learning platform: Which is better?
Although the answer to this question depends entirely on your organization, it’s likely to be the case that buying or licensing a third-party platform is likely to be the better option. It wins when it comes to key determining factors such as cost, time, and features, with the only real downside being that a third-party platform might be relatively inflexible depending on the needs of your organization.Â
With so many platforms and software solutions for data science available on the market nowadays, coupled with the fact that building your own bespoke data science platform will take a relatively long time and require a huge initial investment, it’s hardly surprising that more and more businesses are turning to data science and ML platforms like Qwak and trusting them to do all the heavy lifting when it comes to model development. Â
Why you shouldn’t build your own ML platform
We’re now going to explore why we think that you shouldn’t build your own ML platform. Here’s a quick infographic that you can use as a quick summary:Â
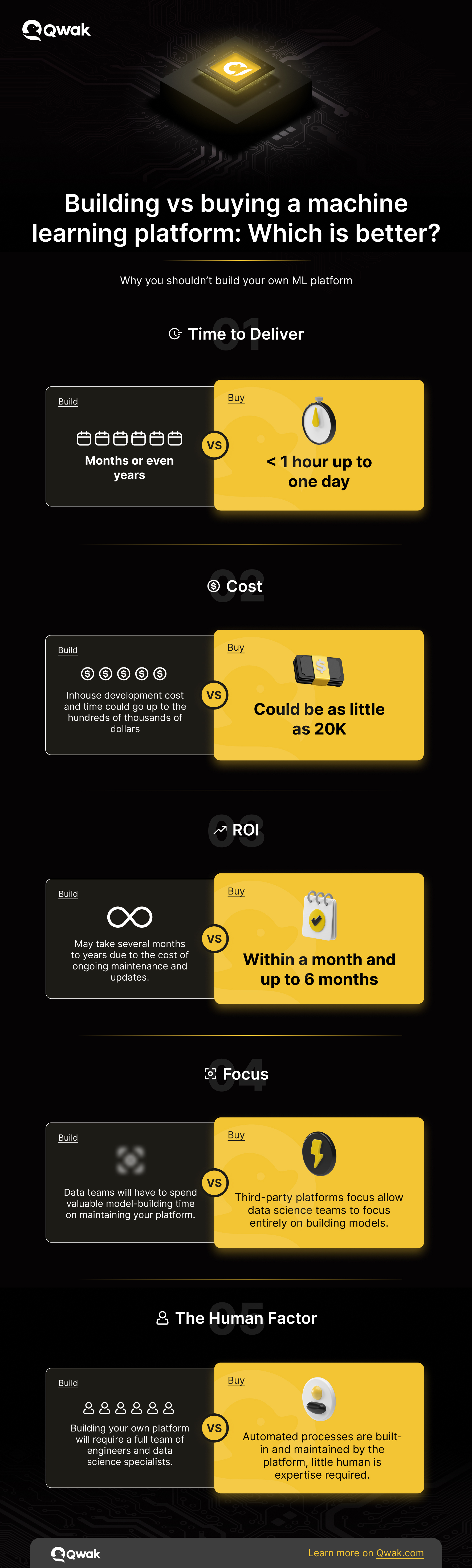
Building requires a lot of time and effort
Data scientists and engineers spend a lot of time developing solutions to support their existing infrastructure in order to accomplish projects. Engineering intensive, non-data science solutions, including things like tracking monitoring, resource management, feature stores, and serving infrastructure, can use up to 65% of the average data scientist’s time. That’s a lot.Â
It’s such a big problem, in fact, that there’s even an “official” term for it—hidden technological debt: the idea that certain necessary work gets delayed during the development of another piece of software or project.This is a common pain point for machine learning teams, and it can take anywhere between six months to a year or longer to build an in-house solution. And that’s not including ongoing maintenance and management which can also drain resources, especially if the in-house solution hasn’t been built robustly enough. It might even require a dedicated team, which is expensive.Â
Building requires an in-house team of specialists
It takes a whole lot of engineering talent to put machine learning into practice. We don’t need to tell you that, though. For any project, each data science team must have an operations team that knows the unique requirements of deploying machine learning models in order to ensure a smooth and seamless workflow.Â
To take care of resources, microservices, clusters, and other things, a typical machine learning team is made up of a combination of specialist engineers and DevOps. With an end-to-end MLOps platform, these processes can be totally automated, which enables operations teams to focus on optimizing and maximizing the use of their infrastructure. More often than not, an organization will decide that outsourcing is a better option than paying to have an in-house team.Â
Although building a bespoke platform can be a wise investment, organizations must have the time and resources available to devote to development without detracting from their existing operations and goals.Â
Building is a lot more expensive
Organizations that decide to try and build their own in-house platform often vastly underestimate the cost of doing so.
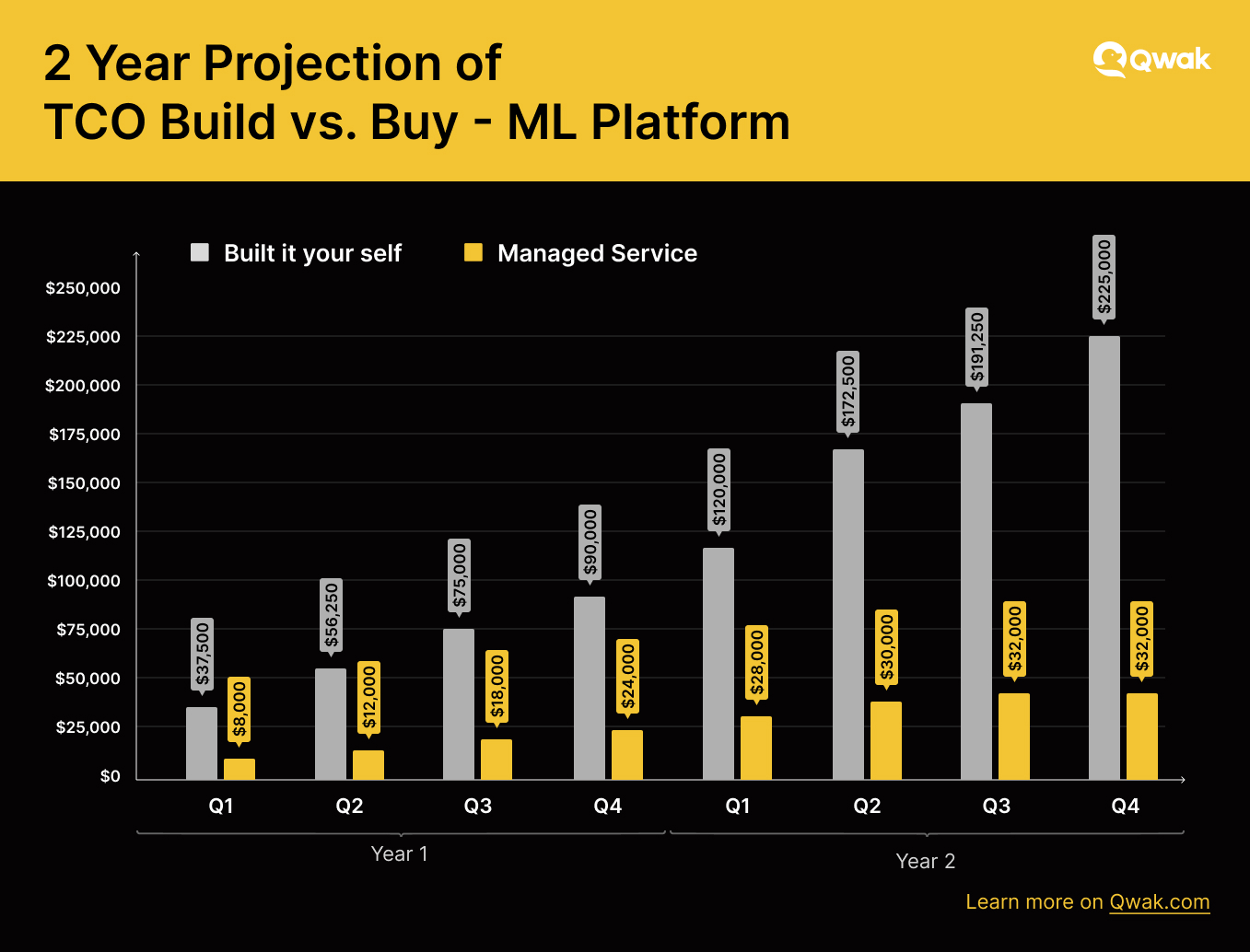
In contrast, the total cost of buying or licensing a third-party platform is a fraction of that when you consider that they are usually created with out-of-the-box MLOps functionality and rapid, limitless scalability in mind. In most cases, firms pay a fixed monthly or annual cost for unlimited access to extremely powerful tooling.Â
Building takes longer to profit from
It can easily take longer than a year to build a functioning ML infrastructure, and it can often take longer to build a data pipeline that is in a position to produce value (i.e., profit) for your organization. Large organizations like the typical FAANG ones have dedicated years, huge budgets, and massive teams to scale and maintain their own platforms to remain competitive. For most, however, this isn’t feasible (and, often, is unnecessary anyway).Â
As we’ve already mentioned, there’s no shortage of fantastic ML platforms and tooling that can offer everything that you need to operationalize your machine learning quickly, efficiently, and cheaply, allowing you to realize a profit much faster than you ever would be able to when building your own solution.
Building takes valuable time away from model development
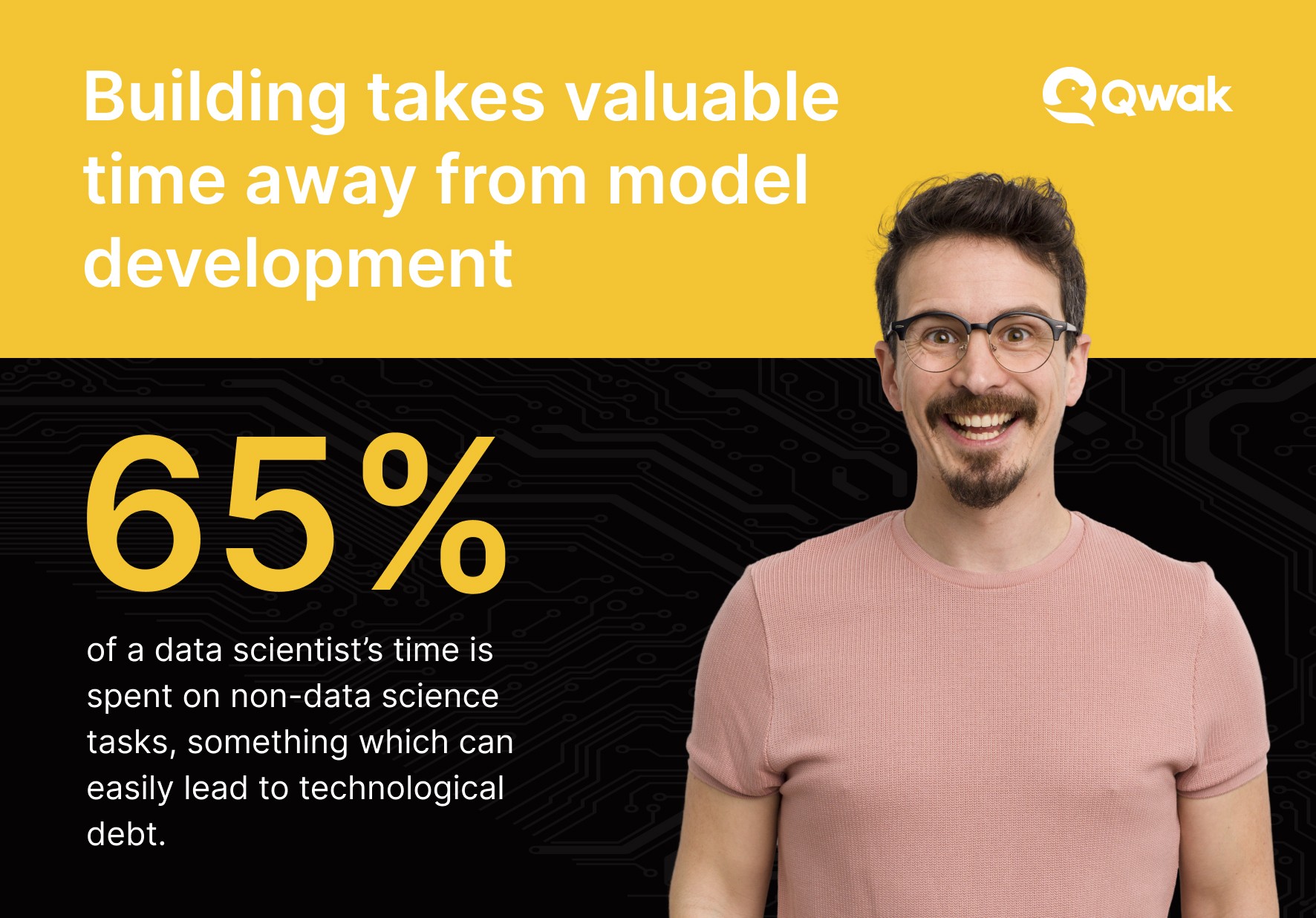
Finally, there’s a certain opportunity cost when it comes to building your own ML platform. As we mentioned earlier, 65 percent of a data scientist’s time is spent on non-data science tasks, something which can easily lead to technological debt. Â
When you’re using a third-party platform like Qwak, however, your data and engineering teams can focus 100 percent effort on the things that matter and that they were hired to do, such as your own data tasks and ML model development workflows. Adopting an end-to-end MLOps platform delivers a considerable competitive advantage that enables your machine learning development to scale massively—the only limit is yourself.Â
Qwak — a leading tool for ML operationalization
Qwak is the full-service machine learning platform that enables teams to take their models and transform them into well-engineered products.Â
Our cloud-based platform removes the friction from ML development and deployment while enabling fast iterations, limitless scaling, and customizable infrastructure.
‍Want to find out more about how Qwak could help you deploy your ML models effectively? Get in touch for your free demo!