
Skip Complex & Costly MLOps
Chosen by the world's best ML team
Why SageMaker users migrate over to Qwak
Using SageMaker, you’re forced to use multiple AWS tools like S3, Docker, Airflow, Jenkins, Cloudwatch, and more to hit the ground running with your models.
With Qwak, the only thing you need is Python ML Libraries to build, train, deploy, and automate your models seamlessly.
Don’t just take our word for it
OpenWeb, a social engagement platform that builds online communities around better conversations, needed a way to scale their expanding data science team’s efforts and show immediate value. Qwak enabled OpenWeb to seamlessly deploy their models quickly and without having to deal with maintenance or infrastructure configuration.
Read case study
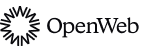
Qwak is the fastest growing Sagemaker Alternative
Feature | ![]() | |
---|---|---|
Time to Live Model | Quick | Slow |
Intuitive UI | V | X |
Zero-config model build & deploy | V | X |
Data Source Integration | Snowflake, MongoDB, BigQuery, Athena, Redshift and more | AWS data sources |
Multi-Cloud support | AWS, GCP | AWS |
Support | 24/7 by ML engineering experts | Standard AWS support |
Designed with a user-friendly interface, Qwak aims to make the MLOps process as straightforward as possible. The platform is built to be intuitive, allowing users to focus on machine learning tasks without the distraction of complex configurations.
SageMaker, though powerful, demands a solid grasp of AWS and engineering expertise. Its UI is less intuitive than specialized platforms, requiring navigation and expertise through multiple AWS services.
A powerful end-to-end ML platform that works for you

Qwak simplifies data pipelines creations through feature sets.

Model infrastructure and data monitoring allow users to easily set up alerts and automations.

A/B testing, variable traffic split and gradual deployments are all supported within Qwak.
What does SageMaker actually cost you?
Supporting multiple models requires a major investment of time, money and maintenance:
- Setup expenses can reach up to $1M.
- Ongoing maintenance costs add up to an additional $500K yearly.
Don’t just take our word for it
A key aspect of Lili’s business strategy is harnessing data and machine learning to enhance customer solutions. This case study explores how Qwak, hosted on AWS, empowers Lili to leverage advanced machine learning capabilities and drive better customer outcomes.
Read case study
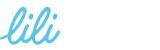