Spot by NetApp: Cloud automation and optimization enhanced to deliver cost fluctuation predictions
Spot by NetApp transforms cloud operations through innovative machine learning and analytics, automating and optimizing infrastructure for optimal, scalable, and cost-effective performance. The platform maximizes cloud investments with cutting-edge technology and predictive insights into infrastructure and cost fluctuations.
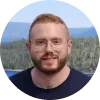
Challenges
- Deliver models to production efficiently and in a timely manner
- Enhance Data Science version deployment with less engineering and/or DevOps dependencies
- Test model versions and feature creation without impacting production environments
- Centrally monitor model performance metrics and control changes. Align feature data pipeline

Implementation
Solutions
Qwak Build: Standardizes an ML delivery project structure This allows every team member to receive the same code structure, data versioning and testing capabilities for every model version.Using Qwak Build increases team members' confidence that new versions won't break any production service and ensures the ability to rollback to previous versions at any time.
Qwak Hosting: Allows Spot’s team to deploy a new real-time model with a click of a button and automatically support Spot’s deployment strategy.
Model Serving: Allows Spot’s team to deploy a new real-time model with a click of a button and automatically support Spot’s deployment strategy which is to deploy a canary version that only a subset of users gets as the first part.
Model Monitoring: Stores all the inference (prediction) data automatically and provides the ability to query real production data at any stage.
Feature Store: Enabled Spot’s data science teams to manage their own data pipeline while writing and scheduling their own features, eliminating the dependency on engineering teams. By using the features built within the feature store the team was able to train their models and serve them with ease. Moreover, the team now has visibility of their feature freshness, lineage and one simple catalog that allows us to easily connect features to models.