MLOps best practices for Generative AI
The rise of foundation models, generative AI, and LLMs are indicating one thing: businesses are turning to data science, machine learning and AI to create a bigger impact and more customer value.
Adapting to fast market shifts brings operational challenges, which organizations need to solve in order to maintain relevance.
Whether you’re building the next ChatGPT, or an ML/AI product that will shake the world, you have to think about:
1. Limiting reliance on external AI APIs and managing your own infrastructure.
2. Fine-tuning models with proprietary data for your specific use cases.
3. Improving models based on user feedback and model outputs.
4. Monitoring model performance and costs in production.
Join Qwak’s Product Manager Guy Eshet to learn more about how to apply existing best in class MLOps techniques to build data pipelines, manage experiments and deploy new model versions.
Join upcoming demo
The rise of foundation models, generative AI, and LLMs are indicating one thing: businesses are turning to data science, machine learning and AI to create a bigger impact and more customer value.
Adapting to fast market shifts brings operational challenges, which organizations need to solve in order to maintain relevance.
Whether you’re building the next ChatGPT, or an ML/AI product that will shake the world, you have to think about:
1. Limiting reliance on external AI APIs and managing your own infrastructure.
2. Fine-tuning models with proprietary data for your specific use cases.
3. Improving models based on user feedback and model outputs.
4. Monitoring model performance and costs in production.
Join Qwak’s Product Manager Guy Eshet to learn more about how to apply existing best in class MLOps techniques to build data pipelines, manage experiments and deploy new model versions.
Learn more from top AI experts
JFrog ML helps companies deploy AI in production



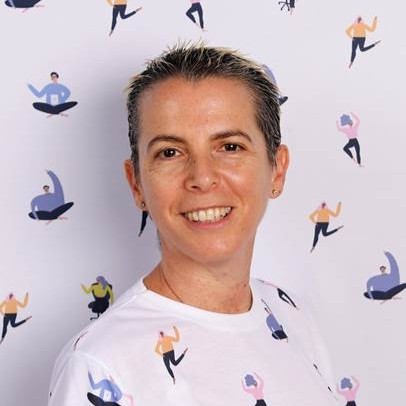
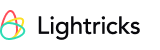