Streaming Aggregation - The Spark Behind Real Time ML
Real time ML tasks, such as credit card fraud detection, recommendation systems, anomaly detection and others use features that are computed as aggregates over real-time data streams.
Data freshness and low latency are key in these use cases, yet achieving them often results in a resource-intensive solution and an angry CFO.
Join Gal Lushi and Yoni Ben-Dayan as they take us through how Qwak’s engineering teams built a turn-key streaming aggregation solution that addresses challenges, while guaranteeing:
- High data freshness, low latency and high throughput
- EXACTLY ONCE and support for late arrivals.
- Efficient handling of multiple small and long time windows.
- Consistency between inference and training (because who wants a training-serving skew?)
Join upcoming demo
Real time ML tasks, such as credit card fraud detection, recommendation systems, anomaly detection and others use features that are computed as aggregates over real-time data streams.
Data freshness and low latency are key in these use cases, yet achieving them often results in a resource-intensive solution and an angry CFO.
Join Gal Lushi and Yoni Ben-Dayan as they take us through how Qwak’s engineering teams built a turn-key streaming aggregation solution that addresses challenges, while guaranteeing:
- High data freshness, low latency and high throughput
- EXACTLY ONCE and support for late arrivals.
- Efficient handling of multiple small and long time windows.
- Consistency between inference and training (because who wants a training-serving skew?)
JFrog ML helps companies deploy AI in production



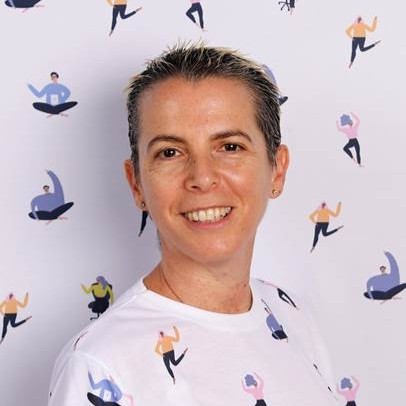
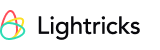